China Creates First Tensor Processor Chip Using Carbon Nanotubes
Chinese researchers have introduced the first-ever carbon-nanotube-based tensor processor chip, marking a major leap forward in AI processing technology. This breakthrough overcomes the constraints of conventional silicon-based semiconductors. The details of this innovation were shared in Nature Electronics on Monday, highlighting a unique systolic array architecture that utilizes the exceptional attributes of carbon nanotube transistors.
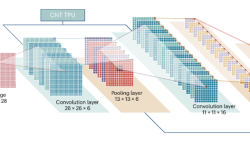
This breakthrough addresses the escalating limitations of traditional silicon-based semiconductors, which are becoming increasingly inadequate for the data processing needs of contemporary AI.
The research team from Peking University detailed their findings on Monday in the journal Nature Electronics, in a study titled "A carbon-nanotube-based tensor processing unit."
The study showcases a novel systolic array architecture that utilizes the attributes of carbon nanotube transistors and tensor operations, offering a potential means to extend Moore's Law, which forecasts the doubling of transistors on a chip approximately every two years.
Current silicon-based computing chips encounter challenges related to size reduction and escalating power consumption. There is a pressing need for new materials that can deliver superior performance and efficiency. Carbon nanotubes, recognized for their excellent electrical properties and ultra-thin structures, are emerging as a viable alternative.
Professor Zhang Zhiyong from the research team highlighted that carbon nanotube transistors outperform commercial silicon-based transistors in both speed and power consumption. These transistors offer a tenfold advantage, facilitating the creation of more energy-efficient integrated circuits and systems, which are critical in the AI era.
While various international research groups have demonstrated carbon-nanotube-based integrated circuits, including logic gates and simple CPUs, this research is the first to apply carbon nanotube transistor technology to high-performance AI computing chips.
"We report a tensor processing unit (TPU) that is based on 3,000 carbon nanotube field-effect transistors and can perform energy-efficient convolution operations and matrix multiplication," said Si Jia, an assistant research professor at Peking University.
This TPU, employing a systolic array architecture, supports parallel 2-bit integer multiply-accumulate operations. Si explained that a five-layer convolutional neural network using this TPU achieved 88 percent accuracy in Modified National Institute of Standards and Technology image recognition tests with a power consumption of just 295 microwatts.
This recent development demonstrates the potential of carbon nanotube technology in high-performance computing and unlocks new possibilities for advancements in AI processing, aiming for more efficient and powerful AI systems.
Sanya Singh contributed to this report for TROIB News