AI in Science: Narrowing the Divide Between Tools and Systemic Innovation
During the 2025 Zhongguancun Forum, a global event that brings together representatives from over 100 countries and features 1,000 speakers, experts delved into a significant paradox: despite AI's transformative impact on scientific tools,...
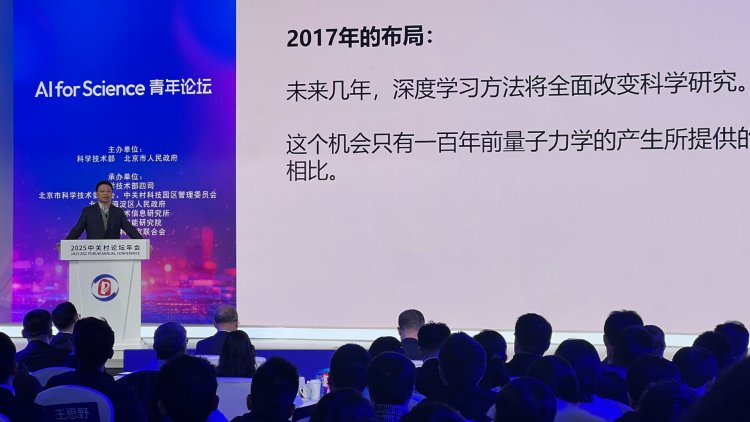
E Weinan, a member of the Chinese Academy of Sciences and a dual-appointed professor at Peking University and Princeton University, highlighted this challenge in his keynote speech.
"We still don't have a proper large language model for science," he remarked during the well-attended AI for Science session. "We are entering an era where we must fully leverage the holistic resources, including the integration of data, computing power, and talents," he added.
Interestingly, there is no direct Chinese equivalent for the term "AI for Science," which underscores its nascent stage despite China’s strategic initiatives in this area, dating back to 2017, as indicated in E's report.
While the term is not new, the latest advancements in large language models (LLMs) have prompted discussions among experts about the potential for a groundbreaking revolution in scientific inquiry, although it has yet to reach its "DeepSeek moment."
Zhou Bowen, director of the Shanghai Artificial Intelligence Laboratory, pointed out that current LLMs have indeed transformed research tools, subjects of study, and the overall research process, yet a true scientific revolution demands comprehensive end-to-end improvements.
Researchers are closely collaborating with engineers and academic institutions. During the session, Zhang Linfeng, founder and chief scientist of DP Technology, unveiled a pioneering platform designed for automated cross-disciplinary experiments.
Operating from Beijing, DP Technology is at the forefront of the AI for Science movement, creating advanced solutions such as the Bohrium Scientific Computing Space Station and the Science Navigator AI platform. These initiatives aim to connect fundamental research with industrial applications, speeding up advancements in drug discovery, materials science, and energy technologies through their innovative integration of AI, physics modeling, and high-performance computing.
"This wave of AI for Science will revolutionize R&D capabilities through systemic augmentation. Such systematic enhancement will break efficiency bottlenecks across pharmaceutical, materials, chemical, and process engineering domains, enabling large-scale, target-driven production of drugs and advanced materials," Zhang explained in an interview.
Additionally, a report released during the event presented the latest iteration of the China AI for Science Innovation Map from the China Institute of Science and Technology Information. This report underscores China's extensive scientific data resources and its ongoing commitment to developing data infrastructure, noting that 20 national scientific data centers are now operational across the country. However, it also points out ongoing challenges related to AI readiness, including high data acquisition costs, inconsistent data formats in engineering, and constraints stemming from data sensitivity in various contexts.
The report further highlights that China and the U.S. are at the forefront globally in terms of co-authored publications and citations within AI for Science, with both countries serving as primary collaborators across various disciplines. It advocates for prioritizing research collaboration in life sciences, earth sciences, and materials science—areas demonstrating the highest levels of global collaborative efforts.
Sanya Singh for TROIB News
Discover more Science and Technology news updates in TROIB Sci-Tech