Catalyst DeepSeek: Unveiling the Secrets to Its Cost-Effective Design
Catalyst DeepSeek: Exploring the Innovative Elements that Drive its Cost Efficiency
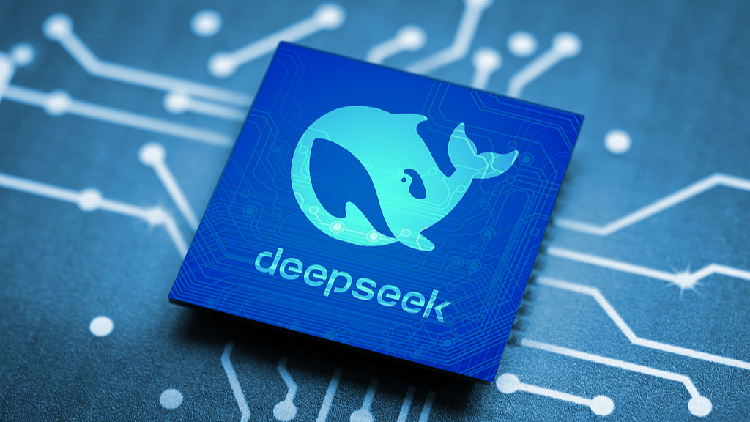
To start with my conclusion: DeepSeek's success hinges on its ability to optimize efficiency within constraints.
Due to restrictions on U.S. chip exports, Chinese enterprises are unable to access advanced AI chips like NVIDIA's H100, which excel in bandwidth and communication speed. This situation has pushed DeepSeek to innovate within the limitations of available hardware, striving for extreme efficiency by reducing computational waste and maximizing the use of every GPU cycle.
Here are some examples of how DeepSeek enhances its performance:
**MoE:** Unlike traditional models such as GPT-3.5 that activate the entire system for every task, DeepSeek's Mixture of Experts (MoE) strategy segments the model into several specialized "experts," activating only those necessary for specific tasks. This significantly boosts efficiency by ensuring that only relevant parts of the model are utilized, thus lowering computational overhead.
**DeepSeekMLA:** This approach reduces memory consumption by concentrating on core contextual information rather than attempting to store every detail. It resembles remembering the essence of a book without needing to recall every single word. The use of latent attention prioritizes essential data, allowing DeepSeek's model to maintain high performance while storing and processing less information.
**Precision Optimization:** DeepSeek opts to store parameters in FP8 instead of higher precision formats like BF16 or FP32, leading to lower memory requirements without a noticeable decline in accuracy. This can be likened to using well-detailed sketches instead of high-resolution images—less data with the same impactful results.
In a technical report on its V3 model, DeepSeek indicated that their training utilized NVIDIA's H800 GPUs. This product was developed as a response to U.S. chip export restrictions affecting China. The H100, a highly powerful GPU for AI training, was off-limits to Chinese companies due to these limitations, prompting NVIDIA to produce a "scaled-down" version, the H800, to align with export regulations.
What does "scaled-down" entail? The main distinction lies in the cross-GPU communication bandwidth. When AI tasks are shared among multiple GPUs, rapid data exchange is essential, similar to a group of workers collaborating. When bandwidth is limited, communication slows, consequently affecting overall computational efficiency. The NVLink bandwidth in the H800 has been markedly curtailed, much like workers transitioning from face-to-face communication to walkie-talkies, which inhibits efficient teamwork.
DeepSeek has chosen to bypass the "commander" role and execute operations independently. NVIDIA provides a GPU computing management system known as CUDA. One can think of CUDA as a factory manager who autonomously assigns tasks to workers, alleviating users from managing minute details. However, DeepSeek discovered that the default scheduling arrangement in CUDA did not meet their extreme optimization requirements given the H800's limitations.
To address this hardware challenge, DeepSeek's engineers opted to sidestep CUDA, controlling the GPUs directly through low-level instructions. They employed a programming method called PTX, allowing for much finer control than CUDA. While this increases the complexity of development, it enables DeepSeek to precisely manage task distribution, thus enhancing the H800's performance and mitigating its bandwidth limitations.
DeepSeek's approach has shown that, despite the constraints of the H800, extreme optimization can still deliver high efficiency in AI training. This suggests that the impact of NVIDIA’s restricted GPUs may not be as substantial as initially expected. Consequently, the market is beginning to reevaluate the feasibility of AI development in China and the potential for reduced reliance on NVIDIA's high-end chips. This shift could play a role in NVIDIA's declining stock prices.
Naturally, there are various reasons contributing to NVIDIA's stock price drop. Beyond DeepSeek's advancements, the market is wary that additional AI companies may seek alternatives to NVIDIA’s ecosystem, considering options from AMD, Intel, and domestic chip manufacturers. DeepSeek's achievements represent not merely a technical milestone, but potentially a signal of change within the AI industry landscape.
**Preview:** In the next article, we will examine how China is fostering global technology competitors—exploring policy, innovation, and the future of artificial intelligence.
**About the author:**
Yang Zhao leads CN's coverage on science, technology, and the environment. He is also the founder of CN's Tech It Out studio, renowned for producing award-winning scientific documentaries including "Human Carbon Footprint," "Architectural Intelligence," and "Land of Diversity."
Anna Muller for TROIB News